The worth of data is essential today more than ever. The way we use data in our respective enterprises directly affects the other vital figures such as revenues, market capitalization, income, and cost. Basically, everything gets affected depending upon the way a company manages its data. Since there can be no operational perfection achieved without data distinction, one needs to ensure that they employ valuable data optimally. A data governance framework is a mandatory requirement for their strategy. Today, we will be touching base with how to create data governance and tips to implement it.
Start with a data maturity archetype
The best method to begin a data governance framework is to employ a data maturity model. This will act as a benchmark using which one can examine their present IT landscape and how aptly they can manage data against their processes, people, technology, or all three of them combined. You can easily find several instances of data maturity models online. The main goal is to create a comparison between the current and the desired state, which is aligned with the goals and aim of the organization. To get a clear perspective on where you are today, you will need to understand what kind of strategies and policies one is required to implement to promote data-driven operations.
Formulating Data Governance Framework
A data governance framework can consist of many small initiatives such as metadata management, master data management (MDM), data quality, or data warehousing. What you will observe is that it doesn’t need to be pushed by one theme. An organization’s data governance framework depends on what the enterprise desires to accomplish with the help of data governance. It is completely up to an organization to concentrate on the theme that will give them the most benefits. These actions can be at a macro or micro initiative level. To understand where to start better, are some key areas that you can focus on:
- Standards and Policies: This kind of program collects standards, examines existing ones, and reviews them against the industrial standards. Another major function it performs is defining the data strategy for an enterprise while facilitating support for the siloed projects attempting to flank the organizational landscape.
- Data Quality (DQ): This type of program helps organizations to find, rectify, and watch out for data quality problems in the system. Such programs generally comprise software that profiles, cleanses, and matches engines. Data Quality initiatives also follow into MDM projects; it defines the master data and provides a 360-degree representation of domains regarding the vendor or customer.
- Data Security and Privacy: Every business needs to adhere to regulations and compliance requirements. This program attempts to address such problems by establishing information security controls, access management rights, data privacy procedures, and more for sensitive data.
- Architecture or Integration: This area of focus aspires to deliver operational efficiency by streamlining data integration architecture aspects which include data modeling, service-oriented architecture (SOA), master data modeling, and others.
- Data Warehouses and Business Intelligence (BI): It supports the application of data warehouses and marts to preserve historical reporting while facilitating futuristic ways of reporting.
- Self-service architectures: It appraised the stewardship and challenges of data preparation. It also facilitates building workflows restricting the paradigm of ‘Shadow IT,’ which occurs very often in enterprises.
Three Steps to Implement Data Governance
A data governance framework coordinates processes, people and technologies collectively to create a standard regarding the management of data as well as the way it is secured and distributed. Since embarking on data governance can feel intimidating at first, here are three ways to get started:
Discover and refine data
The main goal is to overcome data governance challenges by promoting transparency, clarity, and accessibility to the organizational data assets. It is required wherever the data warehouse resides. For instance, it could be within enterprise apps, an SAP, a traditional data warehouse, or a cloud data lake. One needs to make sure there is appropriate data screening so they get the entire rundown of data sources and streams flowing in and departing the company.
Organize trusted data and empower the staff
Organize and streamline the assets for substantial consumption with the help of an extensive data user network that will utilize it in the enterprise.
Data pipeline automation and enabling data access
Once you get data under complete control, you need to extract its value by facilitating it to a broad range of audiences consisting of permitted people and machines. In the digital world, scaling is related to automation. It is necessary to engage people in data governance, but there is always a risk for the same people to turn into a bottleneck. This is why augmentation of the staff’s skills will free them from redundant tasks and ensure that the policies defined by them can be used systematically throughout data flows.
Conclusion
In this blog, we covered the key areas required while developing a data governance framework. Building the framework is crucial, but enforcing it is even more important. A data governance flow is required to be developed on the flanks of the framework to assure progress. Some companies develop a data governance board, which is a specialized unit to control all aspects of it. Once the data governance framework is established being released, it has to be communicated across all channels of the enterprise. To help with this, one needs to relate it with the staff; demonstrating the ways in which such a framework can help accomplish the organizational vision is a good place to start.
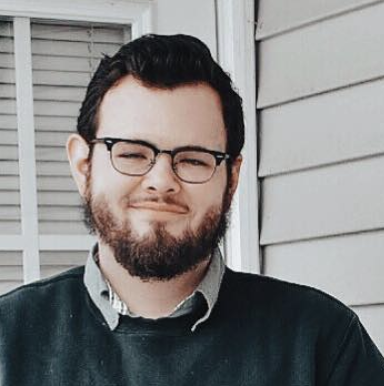
Taylor is a freelance SEO copywriter and blogger. His areas of expertise include technology, pop culture, and marketing.